WGCNA-分析后可以用randomForest包分析hub基因
1、Novel insight on marker genes and pathogenic peripheral neutrophil subtypes in acute pancreatitis https://www.ncbi.nlm.nih.gov/pmc/articles/PMC9444397/
https://www.jianshu.com/p/d7a6e2bb9e23
急性胰腺炎中免疫细胞亚型和可靠生物标志物的鉴定
包:randomForest package
在免疫细胞类型浸润分析中,结果表明,随着AP的进展,外周血DCs、单核细胞、巨噬细胞和中性粒细胞呈上升趋势,而B细胞、CD4 T细胞、CD8 T细胞、Treg细胞、T辅助(Th)细胞、Th1细胞、Th2细胞和Tfh细胞呈下降趋势(图4A)。然后,在严重AP组(MSAP&SAP)和对照组(健康对照和MAP)之间执行随机森林分析(图4B)。前30个基因被列出(图4C)。在这些基因中,有10个基因,包括S100A6、S100A9、S100A12、CD63、ITK、CD5、ANXA3、KLF12、TRABD2A和CCR7,被确认具有重要的诊断价值(图4D)。
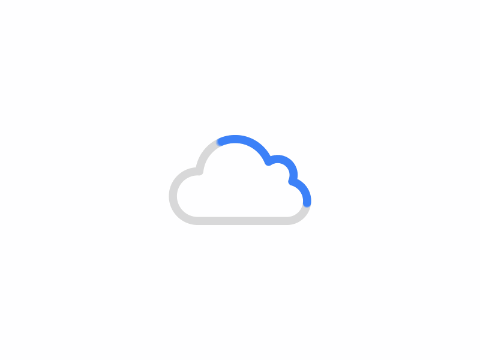
image
2、Machine Learning and Single-Cell Analysis Identify Molecular Features of IPF-Associated Fibroblast Subtypes and Their Implications on IPF Prognosishttps://www.ncbi.nlm.nih.gov/pmc/articles/PMC10778894/
https://mp.weixin.qq.com/s/pfJeJgHamrj1q8saVAE38Q
包:mlr 7种机器学习
3、Integrated Analysis of Single-Cell RNA-Seq and Bulk RNA-Seq Combined with Multiple Machine Learning Identified a Novel Immune Signature in Diabetic Nephropathy https://www.ncbi.nlm.nih.gov/pmc/articles/PMC10258044/
https://mp.weixin.qq.com/s/-3QlJ4UdbW83-x512AVQ1g
包: 4种机器学习 In this study, the RF model, SVM model, AdaBoost model and KNN were developed using the “Random Forest” package, the “e1071” package, the “adabag” package, and the “kknn” package, respectively.
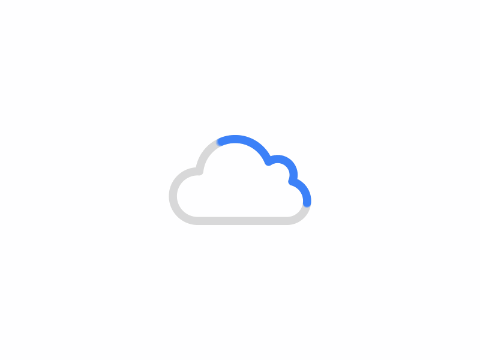
image.png
4、Identification of driver genes in lupus nephritis based on comprehensive bioinformatics and machine learning https://www.ncbi.nlm.nih.gov/pmc/articles/PMC10733527/ https://mp.weixin.qq.com/s/T9UzyjpoDiiHuXO0yRak1g
包:The glmnet package in R was used to conduct the LASSO regression analysis of candidate hub genes in our study.
we utilized the R package “randomforest” to construct our random forest model.
共有 0 条评论