What is information?(续) —— 读A visual introduction to information theory(三)
接着,作者举例,如何将信息论与概率论挂钩起来 —— 经典的就是盒子模型,或者等价的,作者举例的urn模型:
we can consider information theory in the context of a generic random event: repeatedly drawing a marble at random from an urn containing blue, green, yellow, and gray marbles.
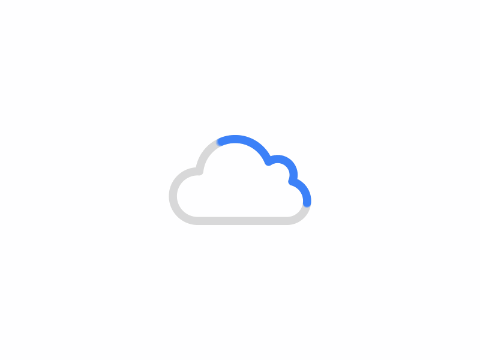
有放回的抽样,用这个来解释信息论:
a) A sequence of two marbles is drawnat random (with replacement) from an urn, giving rise to
b) a probability distribution over the16 possible two-color sequences.
c) Learning that a proposition about the two colors drawn istrue enables the elimination of certain outcomes. For example, learning neither marble is blueeliminates 7/16 possibilities containing 3/4of the the probability mass.
而转换到信息论的角度:
Eliminating probability mass, reducing uncertainty about the outcome, and gaining information are all mathematically equivalent.
如何度量信息(Information gained)呢?
Reduction of 50% of the probability mass corresponds to 1 bit of information....
Each time we can rule out half of the probability mass, we have gained exactly 1 bit of information.
对应的公式是:
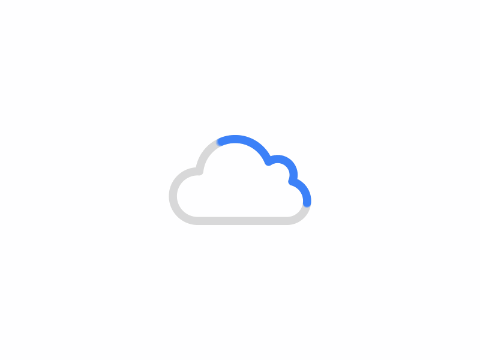
链接完成。
进一步,对于每一个random event,就有:
Since we can calculate the information provided by each outcome, and we know each outcome’sprobability, we can compute the probability-weighted average amount of information provided by arandom event, otherwise known as the entropy.
信息熵的公式是:
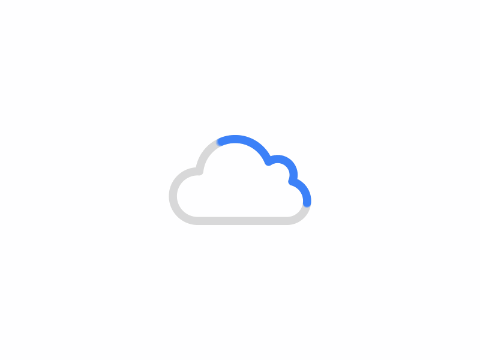
一个有趣的结论是:
It might seem that, since lower probability outcomes contain more information, that randomevents containing many extremely rare outcomes will have the highest entropy. In fact, the oppositeis true: random events with probability spread as evenly as possible over their possible outcomeswill have the highest entropy.
共有 0 条评论