小鬼的m6A图文复现06-样本相关性检验与Peak_Calling
m6A图文复现已经更新到第五期了
群里还是有非常多的小伙伴在问哪个步骤用哪个软件,在这里,我给出一张m6A分析的常规pipeline:
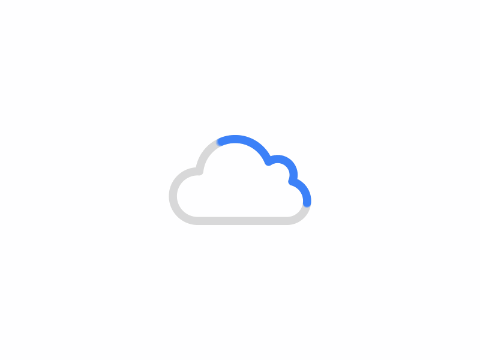
图出处:https://repicmod.uchicago.edu/repic/statistics.php
这个网站集合了非常多的目前已经发表的m6A测序数据,Peak Calling等方法结果以及比较。
1、样本相关性分析
我们前面已经完成了数据比对,在进行Peak Calling之前我们先来看看这几个样本之间的相关性,使用deepTools工具包来看看生物学重复是否聚集到了一起,分组信息为:
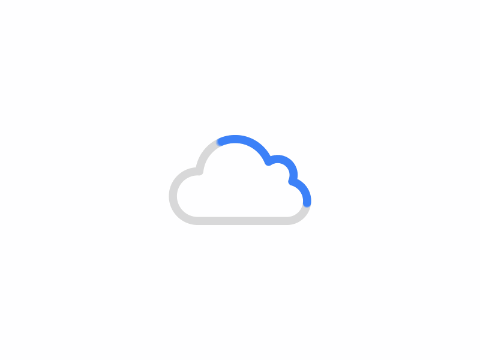
输入数据可以是bam也可以是bigwig,-bs参数默认为10000,可以适当调整这个区间,对相关性计算出来的结果影响还挺大,作者提供的代码用的10,耗时比较久出来结果也不是很好,在原有代码上我还添加了 --plotNumbers 参数在图片上显示相关性大小。
此外corMethod可以选择pearson或者spearman,-o 可以输出为heatmap_pearsonCor.pearson.pdf 的pdf格式或者heatmap_pearsonCor.pearson.png的png格式
# pearson correlation between samples
multiBamSummary bins -bs 1000 -p 10 --bamfiles *bam -o results.npz
plotCorrelation /
-in results.npz /
--corMethod pearson /
--skipZeros /
--plotNumbers /
--labels KO1_MeRIP KO1_NoIP KO2_MeRIP KO2_NoIP KO3_MeRIP KO3_NoIP WT1_MeRIP WT1_NoIP WT2_MeRIP WT2_NoIP WT3_MeRIP WT3_NoIP /
--plotTitle "m6A Correlation across samples" /
--whatToPlot heatmap --colorMap Blues /
-o heatmap_pearsonCor.pearson.pdf --removeOutliers /
--outFileCorMatrix pearsonCorr.rmOut.tab
结果如下,可以看到IP样本聚集在一起,Input样本聚集在一起,WT组和KO组也能看到两个色块。结果还行。
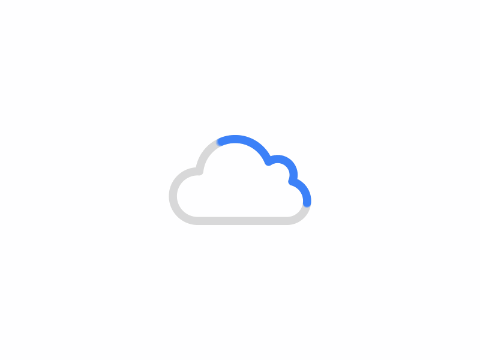
2、Peak Calling
从上面那张流程图里我们可以看到Peak Calling有三个软件:MACS2,exomePeak/exomePeak2,MeTPeak,也是目前市面上m6A分析数据中最常用的三款软件。
关于其中两个软件有篇文献做了测评和比较:
目前对MeRIP-Seq数据进行m6A peak calling分析的软件有两类,一类是早先为ChIP-Seq数据分析所研发的软件,如MACS;另一类则是专门为转录组m6A位点检测而开发的如exomepeak,HEPeak及在前两者基础上发展的MeTPeak。
利用已发表的两份MeRIP-Seq数据,对MACS和MeTPeak两种方法进行了对比,发现尽管MACS可以获得更多的Peak数,但使用MeTPeak所获得m6A Peaks更具有m6A主要发生的位点RRACH(R=G/A; H=A/C/U)这一motif的富集的特点,在MACS 单独发现的Peaks中有超过20%的Peaks分布在TSS这一m6A修饰较少发生的区域。此外,MeTPeak的分析具有链特异性,并且能更好的在剪接的外显子(junction exons)区域发现Peaks,因而在MeRIP-Seq数据分析中,MeTPeak更为适用。
ref:[1] Zeng, Y. , et al. "Refined RIP-seq protocol for epitranscriptome analysis with low input materials." PLoS Biology 16.9(2018):e2006092.
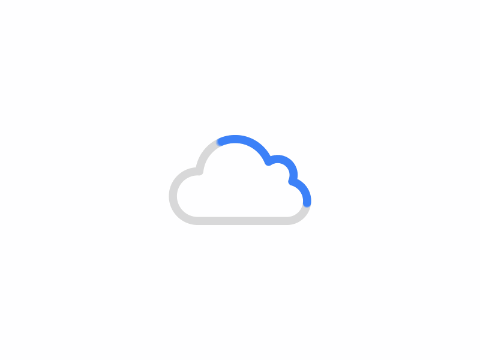
本教程也不太推荐使用MACS2,更加倾向于选择exomePeak/exomePeak2,MeTPeak这些专门为m6A测序数据开发的软件来进行分析。
首先第一个问题,应该也是大多数人会遇到的:Peak Calling的时候有两种做法,分为Sample和Group两种
- 单个样本IP与自身Input进行Peak Calling:这样每个样本就会得到一个Peak结果,对于生物学重复,后面可以合并Peak取交集部分
- 同组的多个IP放在一起,多个Input合并在一起进行Peak Calling:这样一个组就一个Peak结果。
第一种要求每个样本都有自身的Input样本;第二种可以不要求,可以是三个IP,两个Input之类的。
exomePeak2是exomePeak的升级版本,可以参考:孟佳课题组|Peak Calling软件exomePeak以及exomePeak2使用指北,本教程此次使用exomePeak2作为Peak Calling分析。
使用exomePeak2进行Peak Calling
由于exomePeak2为R包,输入数据为bam文件,数据比较大,耗时比较久,代码就写成传参脚本然后在服务器上提交后台运行。
R传参脚本:
rm(list=ls())
options(stringsAsFactors = F)
library(getopt)
spec <- matrix(c(
'help', 'h', 0, 'logical',
'gtf', 'g', 1, 'character',
'bamdir', 'b', 1, 'character',
'ip' , 'i', 1, 'character',
'input' , 'I', 1, 'character',
'paired', 'p', 2, 'logical',
'lib', 'l', 2, 'character',
'mode', 'm', 2, 'character',
'cores', 'c', 2, 'numeric',
'pvalue', 'P', 2, 'numeric',
'od', 'o', 1, 'character'),
byrow = TRUE, ncol = 4)
opt <- getopt(spec)
print_usage <- function(spec=NULL){
cat(' Rscript exomePeak2.R --gtf Mus_musculus.gtf --bamdir Hisat2 --ip ip1,ip2 --input input1,input2 --od ./
Options:
--help -h NULL
--gtf character gtf file, genome annotation [forced]
--bamdir character mapping file, bam dir [forced]
--ip character IP Sample, used for peak calling only
--input character INPUT Sample, used for peak calling only
--paired logical logical of whether the data comes from the Paired-End Library
default: TRUE
--lib character the protocal type of the RNA-seq library, can be
one in c("unstranded", "1st_strand", "2nd_strand");
default = "1st_strand"
--mode character a character specifies the scope of peak calling on genome, can be
one of c("exon", "full_tx", "whole_genome");
Default = "exon"
--cores numeric a numeric value specifying the number of cores used for parallel computing;
default = 6.
--pvalue numeric the cutoff on p values in peak calling
--od character outdir [forced]
/n')
q(status=1)
}
if ( !is.null(opt$help) | is.null(opt$gtf) | is.null(opt$bamdir) ) { print_usage(spec) }
if ( is.null(opt$ip) |is.null(opt$input)) { print_usage(spec) }
if ( is.null(opt$paired) ) { opt$paired <- TRUE }
if ( is.null(opt$lib) ) { opt$lib <- "1st_strand" }
if ( is.null(opt$mode) ) { opt$mode <- "exon" }
if ( is.null(opt$cores) ) { opt$cores <- 6 }
if ( is.null(opt$pvalue) ) { opt$pvalue <- 1e-5 }
if (!file.exists(opt$od)) { dir.create(opt$od) }
library(exomePeak2)
package.version("exomePeak2")
## peak calling for every sample or groups
ip <- unlist(strsplit(opt$ip,split=','))
input <- unlist(strsplit(opt$input,split=','))
ip_bam <- paste0(opt$bamdir,'/',ip,'/',ip,'.Hisat_aln.sorted.bam')
input_bam <- paste0(opt$bamdir,'/',input,'/',input,'.Hisat_aln.sorted.bam')
print(paste('ip_bam:',ip_bam))
print(paste('input_bam',input_bam))
txdb <- GenomicFeatures::makeTxDbFromGFF(file = opt$gtf, format="gtf", dataSource="Ensembl")
if( !is.null(opt$pvalue) ) {
result <- exomePeak2(bam_ip = ip_bam,
bam_input = input_bam,
# data type
paired_end = opt$paired,
library_type = opt$lib,
txdb = txdb,
# cut off
p_cutoff = opt$pvalue,
peak_calling_mode = opt$mode,
# parallel running
parallel = opt$cores, save_dir = opt$od
)
}
## result write out default
服务器提交后台运行:
# 手动造一个config文件,内容为下:
cat group.txt
#MeRIP NoIP NAME
SRR866991 SRR866992 KO1
SRR866993 SRR866994 KO2
SRR866995 SRR866996 KO3
SRR866997 SRR866998 WT1
SRR866999 SRR867000 WT2
SRR867001 SRR867002 WT3
# 生成sh脚本
cat group.txt |awk 'NR>1{print"nohup Rscript exomePeak2.R --gtf Mus_musculus.GRCm38.104.gtf.gz --bamdir mapping/ --ip "$1" --input " $2 " --paired FALSE --lib unstranded --pvalue 1e-05 --od Peak_Calling/"$3 " >Peak_Calling/$3.log &"}' >exomePeak2.sh
# sh脚本内容一行示例如下
nohup Rscript exomePeak2.R --gtf Mus_musculus.GRCm38.104.gtf.gz --bamdir mapping/ --ip SRR866991 --input SRR866992 --paired FALSE --lib unstranded --pvalue 1e-05 --od Peak_Calling/KO1 >Peak_Calling/$3.log &
# 提交后台运行
sh exomePeak2.sh &
下一期分享运行结果以及结果可视化!
共有 0 条评论