生信数据库2: TCGA(RNA-Seq)
TCGA(The cancer genome atlas,癌症基因组图谱)由 National Cancer Institute(NCI,美国国家癌症研究所) 和 National Human Genome Research Institute(NHGRI,美国国家人类基因组研究所)于 2006 年联合启动的项目, 收录了各种人类癌症(包括亚型在内的肿瘤)的各种各样的测序数据,包括RNA sequencing,MicroRNA sequencing,DNA sequencing,SNP-based platforms,Array-based DNA methylation sequencing,Reverse-phase array(包含了基因组、转录组、蛋白组、表观组各个组学数据),另外整合了临床样本信息Biospecimen以及Clinical。
官方网址:https://www.cancer.gov/ccg/research/genome-sequencing/tcga
GDC data portal:https://portal.gdc.cancer.gov/
注意: TCGA数据库中的测序数据共分为四层:level1、level2、level3、level4。其中,level3、level4的数据(经处理及标准化后数据)一般都开放下载的,level1是最原始的数据(Fasta、Fastq等),level2是做了进一步的处理的(经比对Bam文件),这些数据一般是不开放的,需要申请才能下载
1. 数据集介绍
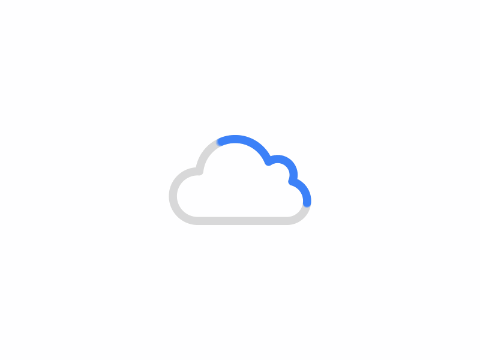
★ "Repository"(存储库页面)是访问 GDC 数据门户中数据的主要方法。它提供了 GDC 中可用的所有案例和文件的概览,并为用户提供了各种过滤器来识别和浏览感兴趣的Cases(案例)和Files(文件)。
1.1
介绍
Files包含与数据文件和实验策略相关的方面,
1.1.1
(数据类别)
ꔷ simple nucleotide variation:简单核苷酸编译
ꔷ sequencing reads:测序读取
ꔷ copy number variation:拷贝数变异
ꔷ structural variation:结构变异
ꔷ transcriptome profiling:转录组分析
ꔷ biospecimen:生物样本
ꔷ dna methylation:DNA甲基化
ꔷ clinical:临床
ꔷ somatic structural variation:体细胞结构变异
ꔷ proteome profiling:蛋白组分析
ꔷ combined nucleotide variation: 核苷酸组合变异
1.1.2
(数据类型)
ꔷ Annotated Somatic Mutation:体细胞突变的注释文件,格式为VCF,采用VEP软件进行注释,文件后缀为vep.vcf.gz
ꔷ Aligned Reads:对齐读取
ꔷ Raw Simple Somatic Mutation: 体细胞突变的原始文件,格式为VCF,文件后缀为vcf.gz
ꔷ Masked Somatic Mutation:open的突变注释文件,免费下载的,格式为MAF,文件后缀为maf.gz
ꔷ Aggregated Somatic Mutation:controlled的突变注释文件,需要账号和权限才可以下载,格式为MAF,文件后缀为maf.gz
ꔷ Gene Level Copy Number Scores:基因水平拷贝数分数
ꔷ Gene Expression Quantification:基因表达量化
等
1.1.3
(实验策略)
ꔷ WXS:全外显子组测序,主要用来检测体细胞突变(SNP),提供了四种方法(MuSE、MuTect2、VarScan2、SomaticSniper)来分析SNP,并分别提供了结果
ꔷ RNA-Seq:全转录组测序,包含lncRNA、mRNA、假基因等等。
ꔷ Targeted Sequencing:靶向测序
ꔷ Genotyping Array:基因分型阵列
ꔷ miRNA-Seq:miRNA测序(miRNA是一类由内源基因编码的长度约为22个核苷酸的非编码单链RNA分子,生物中非常重要的一类非编码小RNA,其在生物体的调控中具有非常重要的作用,在人中大约三分之一的基因受到miRNA的调控),数据只有一种 - BCGSC
ꔷ Methylation Array:甲基化数据(DNA甲基化能引起染色质结构、DNA构象、DNA稳定性及DNA与蛋白质相互作用方式的改变,从而控制基因表达),TCGA提供的甲基化芯片数据,主要以CpG位点为单位,一般认为在基因启动子区域上(基因的TSS的上游2kb到下游500bp之间)的甲基化对该基因的表达会产生影响;目前TCGA提供的公开下载的甲基化数据主要为level3的CpG位点(DNA序列上碱基为C或者G的位点,一般公认的甲基化只会发生在CpG位点上)的甲基化水平的数据
ꔷ WGS:全基因组测序
等
1.1.4
(工作流类型)
ꔷ DNAcopy:DNA拷贝
ꔷ GENIE Simple Somatic Mutation:简单体细胞突变
ꔷ GENIE Copy Number Variation:拷贝值变化
ꔷ BCGSC miRNA Profiling:miRNA分析
等
1.1.5
(数据格式)
略
1.1.6
(平台)
略
1.1.7 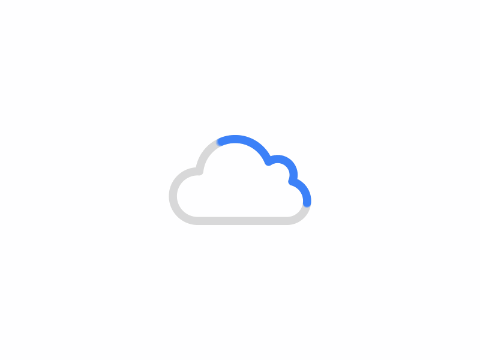
ꔷ controlled:需要申请账号才可以下载
ꔷ open:开放的数据
1.2.
介绍
Cases卡包含与案例和生物样本信息相关的方面
1.2.1
(案例编号)
略
1.2.2
(原发部位)
ꔷ bronchus and lung:支气管和肺
ꔷ breast:乳腺
ꔷ hematopoietic and reticuloendothelial systems:造血和网状内皮系统
ꔷ colon:结肠
ꔷ ovary:卵巢
等
1.2.3
(不同数据集)
ꔷ GENIE
ꔷ FM
ꔷ TCGA
ꔷ TARGET
等
1.2.4
(项目)
ꔷ FM-AD
ꔷ GENIE-MSK
ꔷ GENIE-DFCI
ꔷ GENIE-MDA
ꔷ GENIE-JHU
等
1.2.5
(疾病类型)
ꔷ adenomas and adenocarcinomas:腺癌
ꔷ ductal and lobular neoplasms:导管和小叶肿瘤
ꔷ epithelial neoplasms, nos:上皮性肿瘤
ꔷ squamous cell neoplasms:鳞状细胞肿瘤
ꔷ gliomas:神经胶质瘤
等
1.2.6
(性别)
ꔷ female:女性
ꔷ male:男性
ꔷ unknown:未知
ꔷ not reported:未报导
ꔷ unspecified:不明确
1.2.7
(诊断年龄)
略
1.2.8
(生存状态)
ꔷ not reported:未报导
ꔷ alive:存活
ꔷ dead:死亡
ꔷ unknown:未知
1.2.9
(死亡天数)
略
1.2.10
(人种)
略
1.2.11
(种族)
ꔷ not hispanic or latino:不是西班牙裔或拉丁裔
ꔷ not reported:未报道
ꔷ unknown:未知
ꔷ hispanic or latino:西班牙裔或拉丁裔
2. 数据集下载
2.1 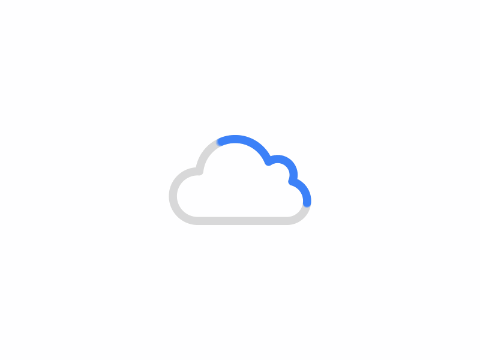
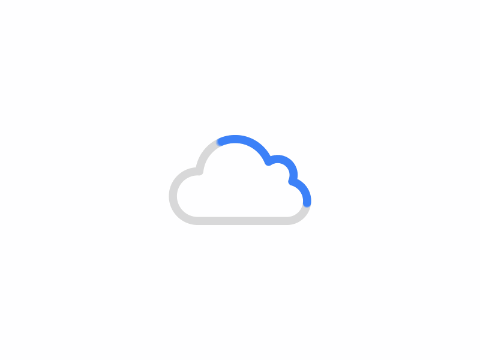
ꔷ Cases:选择"Project - TCGA-LUAD(肺腺癌)"
ꔷ Files:选择"Data Category - transcriptome profiling","Experimental Strategy - RNA-Seq","Workflow Type - STAR-Counts","Access - open"
ꔷ 最终得倒Manifest文件:gdc_manifest_20230608_063108.txt
2.2 下载
工具
TCGA的全部数据都存储在GDC的Data Portal中,如果想要下载大量数据,经常需要使用到gdc官方下载工具:gdc-client
gdc-client工具网址:GDC Data Transfer Tool | NCI Genomic Data Commons (cancer.gov)
$ wget https://gdc.cancer.gov/files/public/file/gdc-client_v1.6.1_Ubuntu_x64.zip
$ unzip gdc-client_v1.6.1_Ubuntu_x64.zip # 解压
$ vim ~/.bashrc # 修改.bashrc文件
# 在最后一行添上:
export PATH=you gdc-client path:$PATH
$ source ~/.bashr c# 激活.bashrc文件
$ gdc-client --help # 检测是否安装配置成功
## usage: gdc-client [-h] [--version] {download,upload,settings} ...
##
## The Genomic Data Commons Command Line Client
##
## optional arguments:
## -h, --help show this help message and exit
## --version show program's version number and exit
##
## commands:
## {download,upload,settings}
## for more information, specify -h after a command
## download download data from the GDC
## upload upload data to the GDC
## settings display default settings
gdc-client download -m gdc_manifest_20230608_063108.txt # 下载数据
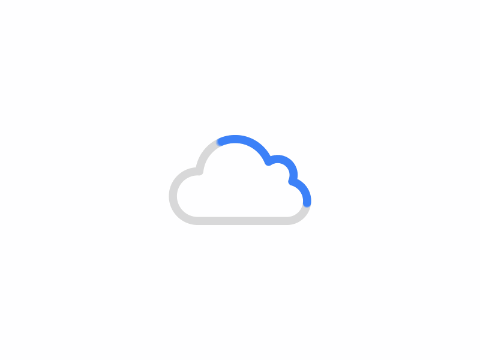
ꔷ 最终得到600个样本(红框,对应600个文件夹)的表达矩阵(绿框 ".tsv文件")
ꔷ Metadata下载json文件即可
2.3 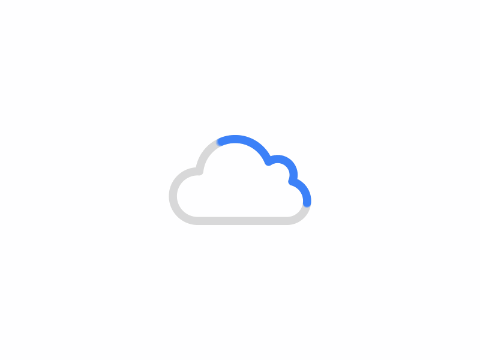
# 读取metadata文件,用于associated_entities和file_name的转换
library("rjson")
json <- jsonlite::fromJSON("/data/shumin/Cibersort/LUAD/metadata.cart.2023-06-08.json")
View(json)
sample_id <- sapply(json$associated_entities, function(x){x[,1]})
file_sample <- data.frame(sample_id, file_name = json$file_name)
# 获取count文件夹下的所有tsv表达文件的 路径+文件名
count_file <- list.files('counts/', pattern = '*.tsv', recursive = TRUE)
#在count_file中分割出文件名
count_file_name <- strsplit(count_file, split = '/')
count_file_name <- sapply(count_file_name, function(x){x[2]})
matrix = data.frame(matrix(nrow = 60660, ncol = 0))
for (i in 1:length(count_file)){
path = paste0('counts/', count_file[I])
data <- read.delim(path, fill = TRUE, header = FALSE, row.names = 1)
colnames(data) <- data[2, ]
data <- data[-c(1:6), ] # 去除前六行注释信息
data <- data[3] #取出unstranded列(第3列),即count数据,对应其它数据
colnames(data) <- file_sample$sample_id[which(file_sample$file_name == count_file_name[I])]
matrix <- cbind(matrix, data)
}
write.csv(matrix, 'LUAD_Ensembl_COUNT_matrix.csv', row.names = TRUE) # 写出文件,此时得到的gene id是"Ensembl ID"
# 设置Gene Symbol为列名
path = paste0('counts/', count_file[1])
data <- as.matrix(read.delim(path, fill = TRUE, header = FALSE, row.names = 1))
gene_name <- data[-c(1:6), 1]
matrix0 <- cbind(gene_name, matrix)
#将gene_name列去除重复的基因,保留每个基因最大表达量结果
matrix0 <- aggregate( . ~ gene_name, data = matrix0, max)
#将gene_name列设为行名
rownames(matrix0) <- matrix0[, 1]
matrix0 <- matrix0[, -1]
write.csv(matrix0, 'LUAD_SYMBOL_COUNT_matrix.csv', row.names = TRUE)
# 分为normal和tumor矩阵
sample <- colnames(matrix0)
normal <- c()
tumor <- c()
for (i in 1:length(sample)){
if((substring(colnames(matrix0)[i], 14, 15) >= 10)){ #14、15位置>=10的为normal样本
normal <- append(normal, sample[i])
} else {
tumor <- append(tumor, sample[i])
}
}
tumor_matrix <- matrix0[, tumor]
normal_matrix <- matrix0[, normal]
3. 差异分析
# 构建分组矩阵
All_sample <- cbind(tumor_matrix, normal_matrix)
# 将数据框里的数据从字符串型转为数值型
All_sample_2 <- as.data.frame(lapply(All_sample, as.numeric))
row.names(All_sample_2) <- row.names(All_sample)
colnames(All_sample_2) <- colnames(All_sample)
# 过滤,原则:有原则是某基因在所有样本中的read counts之和小于样本总数(样本均read counts<1)
All_sample_2 <- All_sample_2[which(rowSums(All_sample_2)/ncol(All_sample_2) >= 1),]
group_list <- c(rep('tumor', ncol(tumor_matrix)), rep('normal', ncol(normal_matrix)))
condition = factor(group_list)
coldata <- data.frame(row.names = colnames(All_sample_2), condition)
3.1
差异分析
library(DESeq2)
# 构建 DESeqDataSet 对象并过滤低丰度数据
dds <- DESeqDataSetFromMatrix(countData = All_sample_2, colData = coldata, design = ~ condition)
keep <- rowSums(counts(dds) >1 ) >= 2
dds.keep <- dds[keep, ]
# 计算归一化系数- sizeFactor
dds <- estimateSizeFactors(dds)
dds_norm <- DESeq(dds.keep)
# 提取结果
result_DESeq2 <- results(dds_norm, contrast = c("condition", "tumor", "normal"), name = 'condition_tumor_vs_normal', alpha = 0.05, lfcThreshold = 2, cooksCutoff = FALSE) # alpha = 0.05可指定padj; cookCutoff是不筛选outliers,因为太多了
summary(result_DESeq2)
##
## out of 36234 with nonzero total read count
## adjusted p-value < 0.05
## LFC > 2.00 (up) : 1566, 4.3%
## LFC < -2.00 (down) : 318, 0.88%
## outliers [1] : 0, 0%
## low counts [2] : 0, 0%
## (mean count < 0)
## [1] see 'cooksCutoff' argument of ?results
## [2] see 'independentFiltering' argument of ?results
result <- as.data.frame(results(dds))
# 提取显著差异表达基因的矩阵
DGE_DESeq2 <-subset(result, padj < 0.05 & (log2FoldChange > 2 | log2FoldChange < -2))
DGE_DESeq2 <- DGE_DESeq2[order(DGE_DESeq2$log2FoldChange), ]
# 输出结果
resOrdered_DESeq2 <- result[order(result$padj, result$log2FoldChange, decreasing = c(FALSE, TRUE)), ] # 默认decreasing = F
resOrdered_DESeq2[which(resOrdered_DESeq2$log2FoldChange >= 2 & resOrdered_DESeq2$padj < 0.05), 'sig'] <- 'up'
resOrdered_DESeq2[which(resOrdered_DESeq2$log2FoldChange <= -2 & resOrdered_DESeq2$padj < 0.05), 'sig'] <- 'down'
resOrdered_DESeq2[which(abs(resOrdered_DESeq2$log2FoldChange) <= 2 | resOrdered_DESeq2$padj >= 0.05), 'sig'] <- 'none'
write.table(resOrdered_DESeq2, 'DESeq2_all.txt', col.names = T, row.names = T, sep = '/t', quote = FALSE)
3.2
差异分析
library(edgeR)
# DEGList对象、构建分组
degs <- DGEList(counts = All_sample_2, group = coldata$condition);degs
# 过滤,删除低表达基因
keep <- rowSums(cpm(degs) > 1 ) >= 2
degs.keep <- degs[keep,]
# 归一化
degs.norm <- calcNormFactors(degs.keep, method = 'TMM')
# 估计离散度
degs.norm <- estimateCommonDisp(degs.norm, verbose=TRUE)
degs.norm <- estimateTagwiseDisp(degs.norm)
# 通过检验差异来鉴别差异表达基因
et <- exactTest(degs.norm)
top <- topTags(et, 50) # 可以设置参数n来调整显示的条目,默认是n = 10;可以通过设置sort.by = "logFC"来按照|logFC|的降序排序;可以通过设置p.value = 0.05来筛选p-adjusted value < 0.05的基因
summary(de <- decideTestsDGE(et, adjust.method = "BH", p.value = 0.05, lfc = 2)) # 默认的参数是adjust.method = "BH",也可以设置为adjust.method = "fdr";p.value = 0.05也是默认的参数,指的是筛选FDR小于0.05的基因;默认的lfc为lfc=0
## tumor-normal
## Down 728
## NotSig 25748
## Up 5413
# 输出结果
resOrdered_edgeR <- et[["table"]][order(et[["table"]]$PValue, et[["table"]]$logFC, decreasing = c(FALSE, TRUE)), ] # 默认decreasing = F
resOrdered_edgeR[which(resOrdered_edgeR$logFC >= 2 & resOrdered_edgeR$PValue < 0.05), 'sig'] <- 'up'
resOrdered_edgeR[which(resOrdered_edgeR$logFC <= -2 & resOrdered_edgeR$PValue < 0.05), 'sig'] <- 'down'
resOrdered_edgeR[which(abs(resOrdered_edgeR$logFC) <= 2 | resOrdered_edgeR$PValue >= 0.05), 'sig'] <- 'none'
write.table(resOrdered_edgeR, 'edgeR_all.txt', col.names = T, row.names = T, sep = '/t', quote = FALSE)
3.3
差异分析
library(Lima)
# 构建design 样本分组矩阵
design <- model.matrix(~0 + coldata$condition)
rownames(design) = colnames(All_sample_2)
colnames(design) <- levels(coldata$condition)
# 构建DGElist对象
DGElist <- DGEList(counts = All_sample_2, group = coldata$condition)
# 去除表达量过低的基因
keep <- rowSums(cpm(DGElist) > 1) >= 2
table(keep)
DGElist <- DGElist[keep, ,keep.lib.sizes = FALSE]
# 计算列的矫正因子
DGElist <- calcNormFactors(DGElist )
# 将count值转化成log2-counts per million (logCPM),准备进行线性回归
v <- voom(DGElist, design, plot = TRUE, normalize = "quantile")
# 对每一个基因进行线性模型构建
fit <- lmFit(v, design)
# 构建比较矩阵
cont.matrix <- makeContrasts(contrasts = c('tumor - normal'), levels = design)
# 构建芯片数据的线性模型,计算估计的相关系数和标准差
fit2 <- contrasts.fit(fit, cont.matrix)
# 基于贝叶斯计算T值,F值和log-odds
fit2 <- eBayes(fit2)
nrDEG = topTable(fit2, coef = 1, n = Inf, adjust = "BH")
nrDEG = na.omit(nrDEG)
# 首先对表格排个序,按 padj 值升序排序,相同 padj 值下继续按 log2FC 降序排序
resOrdered_limma <- nrDEG[order(nrDEG$adj.P.Val, nrDEG$logFC, decreasing = c(FALSE, TRUE)), ] # 默认decreasing = F
resOrdered_limma[which(resOrdered_limma$logFC >= 2 & resOrdered_limma$adj.P.Val < 0.05), 'sig'] <- 'up'
resOrdered_limma[which(resOrdered_limma$logFC <= -2 & resOrdered_limma$adj.P.Val < 0.05), 'sig'] <- 'down'
resOrdered_limma[which(abs(resOrdered_limma$logFC) <= 2 | resOrdered_limma$adj.P.Val >= 0.05), 'sig'] <- 'none'
# 输出选择的差异基因总表
write.table(resOrdered_limma, 'limma_all.txt', col.names = T, row.names = T, sep = '/t', quote = FALSE)
参考:
共有 0 条评论