CS111 Data science
my wechat:Yooo932851
Don't hesitate to contact me
Introduction
• This is the second part of Lecture 9; it covers clustering
• Clustering refers to a very broad set of techniques for finding subgroups, or clusters, in a data set
• Seek to partition observations into distinct groups so that the observations within each group are similar to each other, while observations in different groups are different from each other
• What means to be “di fferent” and “similar”? Often it is a domain specific issue
• Think about why clustering is not classification; recall fraud detection in credit card transactions vs. fraud detection in medicare expense
• Sometimes, people do PCA first and then do clustering in exploratory data analysis (EDA)
• PCA looks to find a low-dimensional representation of the observations that explain a good fraction of the variance
• Clustering observations looks to find homogeneous subgroups among the observations
Different Clustering Approaches
• There exist a great number of clustering methods
• In this section, we focus onK-means clustering andhierarchical clustering
• K-means clustering : partition the observations into a pre-specified number of clusters
• hierarchical clustering (optional): not know in advance how many clusters we want; end up with a tree-like visual representation of the observations, called adendrogram
K-means Clustering
• LetC1, ··· , CK denote sets containing the indices of the observations in each cluster. These sets satisfy two properties:
• C1fi C2··· fi CK ={1, ··· , n}
• Ck andC kÕ have empty intersection, for di fferentk andkÕ
• Idea for k-means clustering: a good clustering is one for which the within-cluster variation is as small as possible
• W (Ck ): a measure of the amount by which the observations within a cluster di ffer from each other, defined by
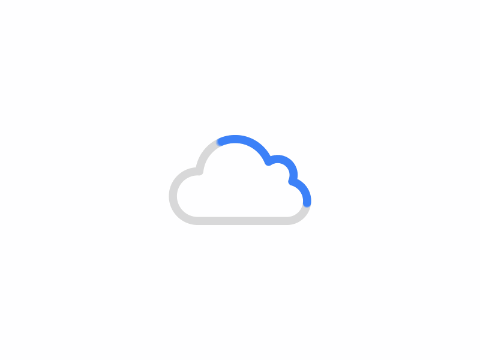
• K-means algorithm solves
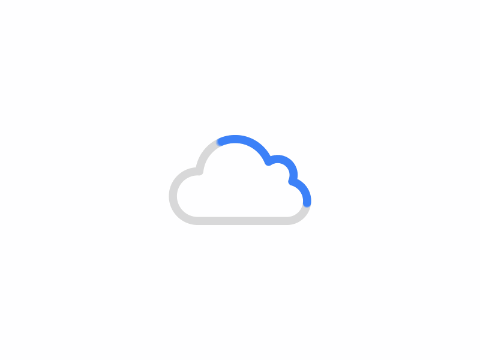
K-means Clustering - an illustration
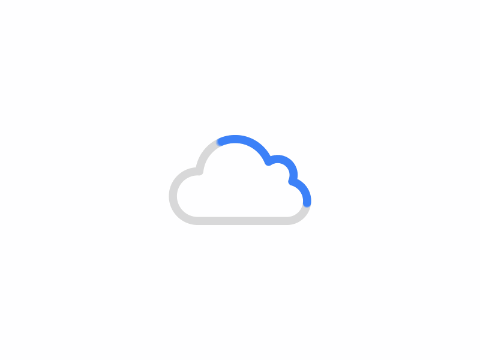
K-means Algorithm
• Brutal force way is infeasible computationally
• The next algorithm finds an approximate solution
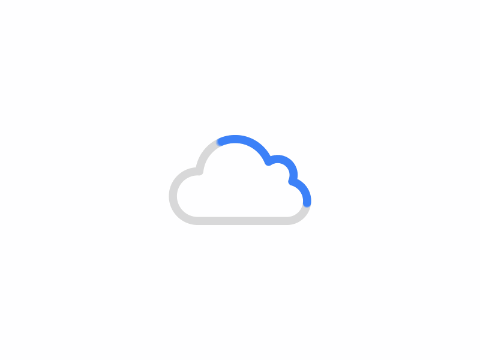
Why the Algorithm Works?
• Algorithm 10.1 is guaranteed to decrease the value of the following objective at each step
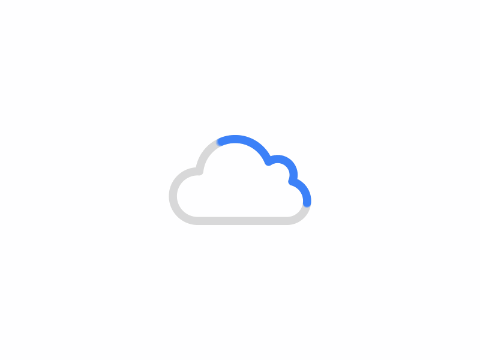
• To understand this, we need
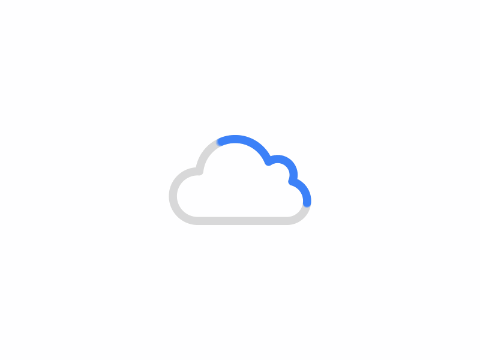
• Because the K-means algorithm finds a local rather than a global optimum, the results obtained will depend on the initial (random) cluster assignment of each observation
• It is important to run the algorithm multiple times from di fferent random initial configurations (which one do you choose in the end?)
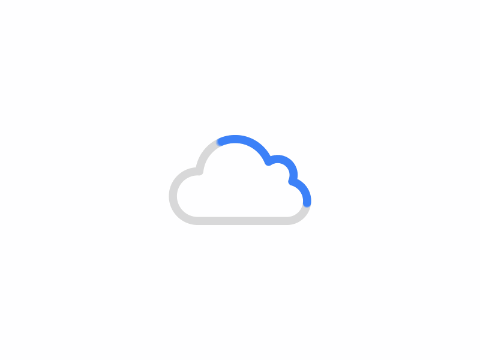
Hierarchical Clustering (Optional)
• Hierarchical clustering is an alternative approach to K-means clustering which does not require that we commit to a particular choice ofK
• In this section, we describebottom-up oragglomerative clustering
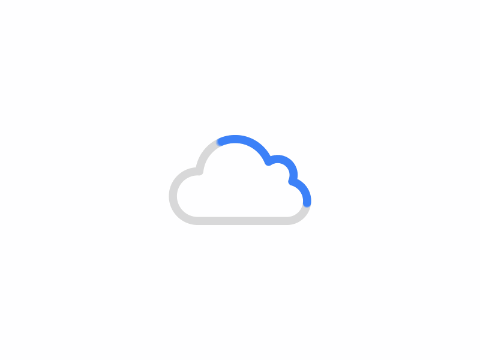
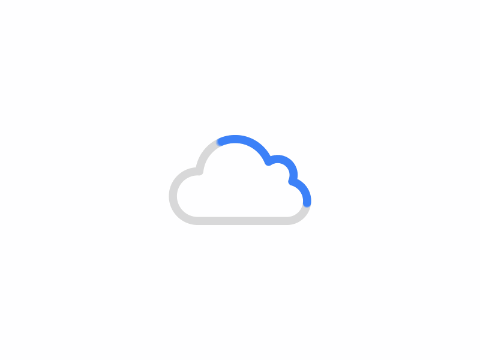
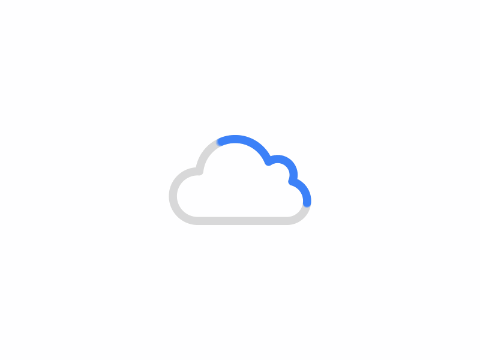
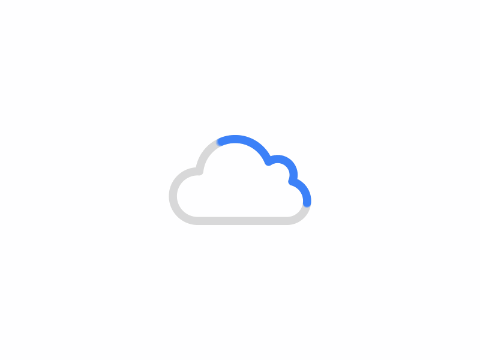
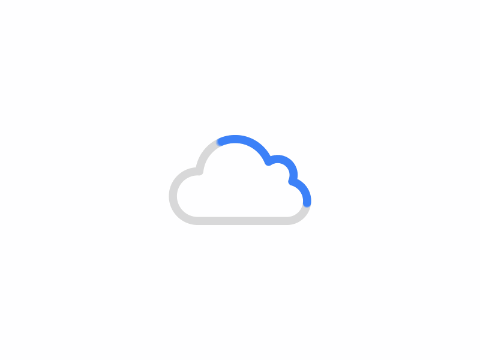
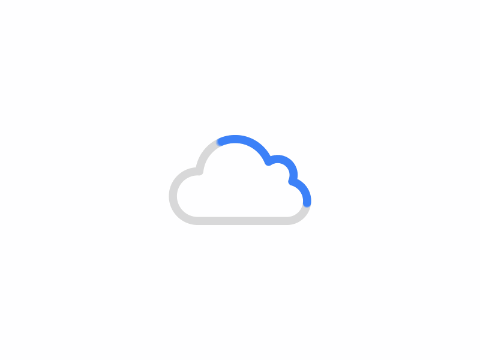
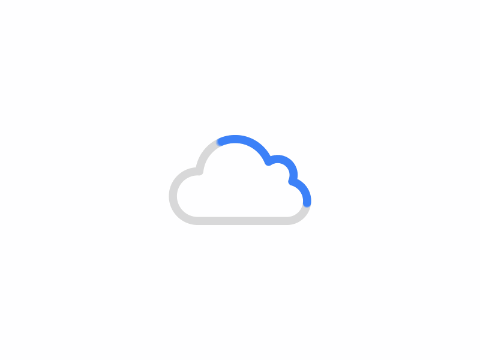
Practical Issues in Clustering
• Should the features first be rescaled in some way?
• In the case of K-means clustering, how many clusters should we look for in the data?
• In the case of hierarchical clustering (optional),
• What dissimilarity measure should be used?
• What type of linkage should be used?
• Where should we cut the dendrogram in order to obtain clusters?
• Answers : With these methods, there is no single right answer–any solution that exposes some interesting aspects of the data should be considered
• In practice, we try several di fferent choices, and look for the one with the most useful or interpretable solution
• Caution about a data set : clustering results should not be taken as the absolute truth
共有 0 条评论